Warning, Warning: Cleanse Data Before and After use
Is your business working with “dirty” data? Are there inconsistencies across your data fields—such as varying date formats, currency symbols, or measurement units?
Incorrect and inconsistent data creates more problems than having no data at all. Inaccuracies lead to faulty decision-making. Data that’s not formatted correctly can also prevent users from running reports to gather information and verifying the status of workflows.
And when your staff works with dirty data, errors can cause them to take actions that ultimately erode customer trust. As data degrades, communications and workflows suffer. Customers may lose confidence and worry your organization does not take good care of their sensitive data.
Conversely, when you properly cleanse data, you improve your capacity to conduct relationship-focused marketing campaigns and your ability to enhance overall customer experiences. Yes, it seems like a lofty goal, but the devil is often in the details. That’s why it’s so critical to cleanse your data before and during use.
What Is Data Cleansing?
Let’s look at data cleansing in terms of banking. In banking and many other business sectors, staffs need to cleanse CRM, ERP, and Core data to run reports daily and maintain customer accounts. The cleansing process needs to occur when first opening data files and on an ongoing basis.
Reports and current dashboard data can also assist in making sure the bank follows regulations related to managing customer accounts. In addition, data impacts a wide range of other banking workflows:
- Tracking customer point of origin
- Establishing debit cards
- Changing account profiles
- Distributing tax forms
- Distributing statements and notices
- Maintaining credit bureau data
- Conducting marketing campaigns
- Adding new accounts
- Setting up third-party systems
- Closing accounts
- Generating reports and visuals
- Tracking financial trends
As part of the data cleansing process, you also need to eliminate duplicate data, which can impact multiple workflows. Cleansing also facilitates running reports to make sure the bank follows regulations in managing customer accounts.
Ultimately, cleansing fixes inaccurate and incomplete data in a dataset. By removing errors, you improve the quality of your data and increase the efficiency of your workflows as well as your reporting. With accurate data, your staff completes tasks faster, and your management team makes business decisions quickly with confidence.
Using Robots to Automate Data Cleansing
Cleansing data can be executed manually, semi-automatically, or with full automation—using Robotic Process Automation (RPA) bots. Bots simulate manual work and can be programmed to take the same steps as a human, given certain logical circumstances. These include tasks such as filling in forms, moving files, tracking changes, and kicking off other programs.
RPA bots follow a pre-defined logic based on the circumstances that may occur. Many RPA bot flows only contain recorded keystrokes while others have complex logic statements built into the before and after clicks.
You can kick off bot tasks manually or by using scheduling tools. Bots do not tire out—they can run 24 hours a day, 7 days a week without one single snack break. They don’t get bored, they don’t check their social media posts, and they don’t fall asleep at the job.
The use of bots also increases efficiency, reduces the cost of data entry, and allows for integration with workflow software and other technical solutions. Return on investment calculations are easy to obtain, despite the sometimes-high cost of RPA software. The good news is that with the introduction of Microsoft Power Automate, the cost of the RPA bot flows blows the ROI OFF THE CHARTS!
Reducing the Risk of Your Data Cleansing Process
Just like any other project where data is involved, the implementation of a risk management process for data cleansing is critical. A well-thought-out and tested plan reduces risks and the overall length and number of issues for any project.
To take on this challenge, the ATX team begins by training and receiving input from customer employees. We then plan the data sets and the bot flows based on known issues and the feedback. From there, we thoroughly test the data sets, document the process, and implement the project. This includes testing in the live environment.
Establishing Data Standards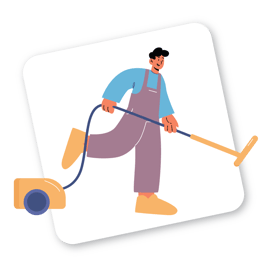
In addition to using technology to cleanse your data, developing standards can guide your staff to open and handle files correctly. This ensures they don’t add extra or incorrect data when executing a workflow.
Standards play a key role because a lack of functional data standards can create confusion. Errors include shipping products to the wrong location and losing track of customer tax IDs.
To establish and evaluate data standards, follow this four-step process:
1. Establish data entry guidelines to describe how to handle data, spelling, and punctuation. For example, if a customer is an LLC, how do you input an LLC into the system? How do you show the relationships between customer contacts and the customer’s business profile? The standards should be simple enough for everyone to understand but complex enough to provide guidance when encountering an unusual situation. Consistency is the key when it comes to reusing and referring to data for reporting.3. Automate corrections. This is where robotic process automation or using scripts comes in handy. They speed up data entry and make the data entries consistent.
Once the standards for data input are defined, the workflow process can then serve as a path for automation and facilitate complex tasks.
Assuring Data Quality
Across all industries, maintaining data by using staff designated for keeping the standards will become more and more expensive as a company grows. The efficiency of the manual approach decreases further as a company adds assets and customers. Fixing these issues is complex but simple to resolve if reporting and automation are in place.
Implementing a fix is vital because dirty data may seem like a bad joke, but it’s just bad for business. Lacking data standards and with no automated way to clean up items, there is little that can be done—other than brute force changing the data to keep it clean.
To learn more about how to cleanse data to ensure processes flow efficiently and reports generate accurate information, contact ATX today.
Author: Denise Butler